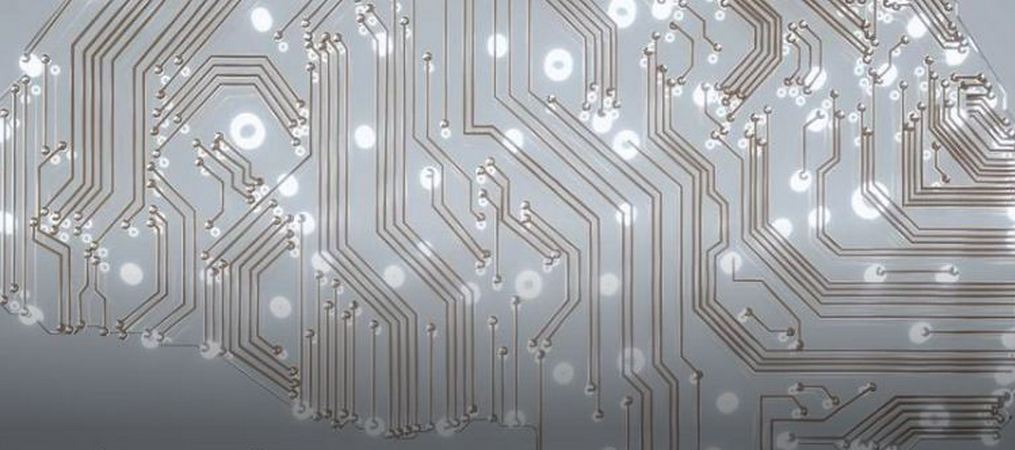
Using Machine Learning to Improve Student Success in Higher Education
Many higher education institutions now use data and analytics as an integral part of their processes. Thoughtful application of machine learning and advanced analytics can support success and overall student experience. Whether the goal is to identify and support pain points in the student journey, more efficient access to resources, or improve student and school experiences, institutions see the benefits of data-backed solutions.
Those at the forefront of this trend are focusing on harnessing analytics to increase program personalization and flexibility, as well as to improve retention by identifying students at risk of dropping out and reaching out proactively with tailored interventions.
For example, institutions that use rule-based linear approaches look at indicators such as low grades and low school attendance to identify students at risk of dropping out of school; institutions then reach out to these students and launch initiatives for better support. Although such initiatives may be beneficial, they are often implemented too late and target only a subgroup of the at-risk population. This approach could be a well-improvised solution to two problems facing student success leaders at universities. First, there are several variables that could be analyzed to indicate the risk of exhaustion (such as academic, financial, and mental health factors and sense of belonging). Second, linear rules-based approaches may fail to identify students who, for example, may have decent grades and above-average attendance, but who struggle to deliver their assignments on time due to other difficulties.
Advanced analytics in action: How institutions have improved enrollment, retention and, ultimately, student experience
One private university had recently enrolled its largest freshman class and was looking to increase its enrollment again. The institution wanted to both reach more prospective first-year undergraduate students who would be a great fit for the institution and improve conversion in the enrollment journey in a way that was manageable for the enrollment team without significantly increasing investment and resources.
For this institution, the modeling of advanced analytics had an immediate impact. The initiative suggested future opportunities for the university to enroll more freshmen with greater marketing efficiency. When initially tested (before the application deadline), the model accurately predicted 85 percent of applicants who applied, and predicted 35 percent of candidates at that point in the cycle most likely to enroll, assuming no change in admission criteria. The enrollment management team is now able to better prioritize its resources and time on high-potential leads and applicants to yield a sizable class. These new capabilities will give the institution the flexibility to make strategic choices; rather than focus primarily on the size of the incoming class, it may ensure the desired class size while prioritizing other objectives, such as class mix, aid allocation, or budget savings.
Source: www.mckinsey.com